Urban Computing: Transforming Air Quality in Smart Cities
- Yash Bhavsar
- Mar 10
- 10 min read
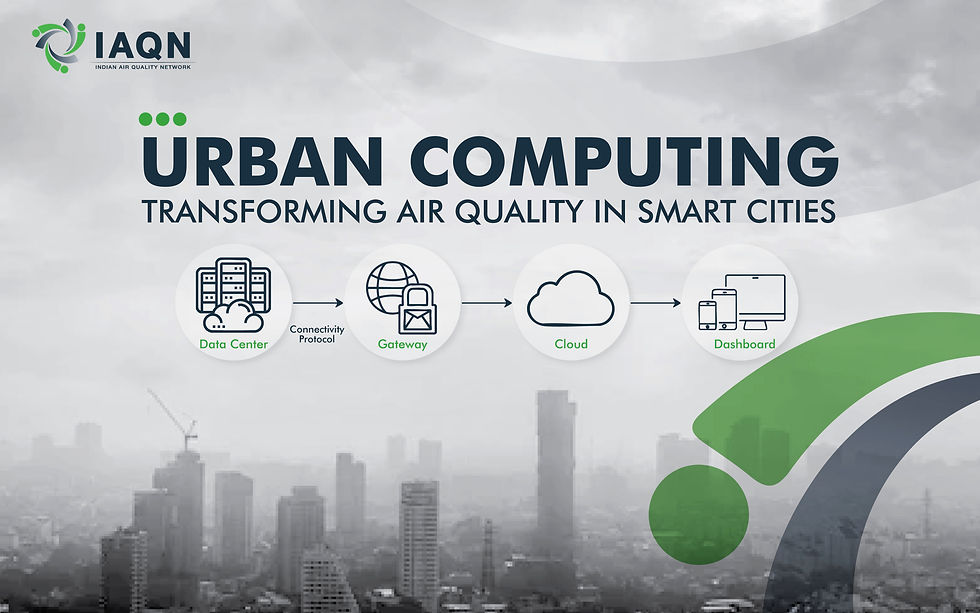
Have you ever wondered how cities can tackle air pollution before it becomes a crisis? That’s where urban computing comes in. It uses smart sensors, AI, and data analytics to track and improve air quality in real-time. Imagine a city covered with tiny air quality sensors, constantly collecting pollution data. This information is sent to AI-powered systems that analyze patterns, predict pollution spikes, and help city officials take action. Satellite imaging and GIS mapping make it even smarter by pinpointing pollution hotspots and helping plan better roads, green spaces, and traffic routes.
With urban computing, cities can control industrial emissions, manage traffic to cut pollution, and even send real-time air quality alerts to citizens. But there are challenges, too. Data accuracy, sensor maintenance, and privacy concerns need to be addressed. So, what’s next? More advanced AI models, better pollution prediction, and secure data-sharing technologies are shaping the future of clean air. Cities worldwide are adopting these innovations to create healthier, safer environments for everyone. In this blog, we’ll explore how urban computing is making the air cleaner, the challenges it faces, and what’s coming next. Let’s dive in!
Understanding Urban Computing and Its Role in Air Quality
Cities are facing a growing air pollution crisis, as air pollution is identified as a top global risk. Urban computing is helping tackle this issue by using smart technology, data, and AI to monitor and improve air quality in real-time. The miniaturization of sensing, computing, networking, and communication technologies in urban spaces has led to the development of new technological ecosystems for smart cities.
At its core, urban computing connects IoT sensors, cloud platforms, and AI-driven tools to track pollution levels. Small air quality sensors placed across cities collect data on pollutants like PM, NO₂, CO, and VOCs. This data is processed using AI and cloud computing, helping scientists and policymakers identify pollution trends and predict future spikes. GIS and satellite imagery add another layer by mapping pollution hotspots and guiding city planning efforts.
One of the biggest advantages of urban computing is AI-powered pollution forecasting. By analyzing weather, traffic, and industrial activity, machine learning models can predict pollution spikes before they happen. Cities can then take preventive steps, like adjusting traffic flow to reduce emissions or issuing public health warnings.
However, challenges remain: sensor accuracy, data security, and system reliability need improvement. Future innovations like edge computing for faster data processing and blockchain for secure pollution data tracking will make urban computing even more effective. By integrating smart technology into air quality management, cities can take action before pollution becomes a crisis, leading to healthier urban environments for all.
Key Technologies Powering Urban Environmental Monitoring
As air pollution intensifies, urban computing transforms how cities monitor and manage air quality. Advanced IoT sensors, big data analytics, AI, and GIS mapping enable real-time pollution tracking and predictive interventions.
IoT Sensors: Real-Time Air Quality Monitoring
IoT-based air quality sensors continuously collect data on pollutants like PM1, PM2.5, PM10, NO₂, CO, SO₂, O₃, and VOCs. These sensors are low-power, cost-effective, and scalable, allowing dense deployment across cities. They transmit real-time data to cloud platforms using LoRaWAN, NB-IoT, or 4G, enabling instant pollution analysis. This data helps authorities enforce pollution control measures, issue public health alerts, and optimize city planning. However, sensor calibration and standardization remain key challenges for ensuring accuracy.
Were you aware of this? It is estimated that air quality monitoring IoT products and services will have an economic value between 50 and 60 billion U.S. dollars by 2025, and this range is expected to grow to 60 to 70 billion U.S. dollars by 2030.
Sensor Technologies Used in Smart Cities
Sensor Type | Pollutants Measured | Advantages | Limitations |
Electrochemical Sensors | NO₂, CO, O₃, SO₂ | Low cost, portable | Prone to drift, requires calibration |
Laser-Based Optical Sensors | PM1, PM2.5, PM10 | High precision, real-time response | Sensitive to humidity |
Metal Oxide Sensors (MOS) | VOCs, NH₃, NOx | Compact, robust | Cross-sensitivity issues |
NDIR (Non-Dispersive Infrared) | CO₂ | Accurate for indoor air | Costlier than MOS |
Big Data and AI for Pollution Prediction
Data collection is the first and the most essential part of the analysis and prediction. For the air pollution prediction, the data can be traffic data (fuel consumption, age of the vehicle, emission rate, and traffic volume), Environmental data (pollutant concentration, humidity, wind speed, wind direction, etc.), Geographical Data (longitude, latitude, land use, etc.), Socioeconomic Data (population density and rate of urbanization).
Big data and AI process vast amounts of environmental, meteorological, and vehicular emission data to identify pollution patterns and predict air quality trends. Machine learning models analyze weather conditions, industrial emissions, and traffic congestion to forecast pollution hotspots. AI-powered pollution forecasting systems help authorities take proactive measures, such as traffic diversions, industrial emission regulation, and public advisories. Cloud computing further enhances this by enabling scalable storage and real-time analytics for urban environments.
Key AI Techniques in Pollution Forecasting
Time-Series Forecasting with LSTMs (Long Short-Term Memory Networks):
Used to predict pollution trends by analyzing historical AQI, meteorological conditions, and emissions data.
China’s Blue Sky Project uses LSTMs to anticipate NO₂ and PM2.5 spikes up to 72 hours in advance, allowing officials to enforce traffic restrictions and temporary industrial shutdowns.
Graph Neural Networks (GNNs) for Spatial Air Quality Modeling:
GNNs map pollution dispersion across cities, integrating sensor networks, GIS data, and traffic patterns.
Barcelona’s Urban Air Project employs GNN-based models to identify high-risk zones and recommend optimal locations for green infrastructure.
Hybrid AI Models (Fusion of ML & Physical Models):
Combines machine learning with physical atmospheric dispersion models (e.g., WRF-Chem, CMAQ).
Researchers at MIT developed an AI model integrating satellite-derived aerosol data with meteorological simulations, improving urban PM2.5 predictions by 40%.
GIS and Satellite Imaging for Environmental Mapping
Remote sensing is a powerful tool in computer science. It has proven revolutionary for environmental monitoring and management.
Geographic Information Systems (GIS) and satellite imaging play a crucial role in monitoring, analyzing, and mitigating urban air pollution. These technologies provide spatial intelligence, allowing authorities to identify pollution hotspots, track emission sources, and design effective mitigation strategies.
High-resolution satellite imagery, combined with LiDAR (Light Detection and Ranging) and remote sensing techniques, enables real-time air quality monitoring. By analyzing aerosol optical depth (AOD) data, scientists can estimate PM2.5 and PM10 concentrations over large geographic areas. Multispectral and hyperspectral imaging further enhances pollution detection by identifying specific gases such as NO₂, CO, and SO₂.
GIS platforms integrate satellite data with ground-based sensor readings to create dynamic pollution maps. These maps help policymakers visualize pollution trends, assess land use impacts on air quality, and optimize urban planning for better ventilation and green spaces. Additionally, spatial modeling and predictive analytics allow cities to forecast pollution dispersion and implement targeted interventions.
Satellite-Based Air Quality Monitoring:
Sentinel-5P (ESA): Tracks NO₂, SO₂, CO with 7 km resolution.
MODIS (NASA): Estimates PM2.5 at global scales.
TROPOMI: High-resolution mapping of urban air pollutants.
How Urban Computing Improves Air Quality in Smart Cities
Urban computing is transforming air quality management by integrating IoT, AI, and big data into urban infrastructure. Smart cities leverage real-time data, predictive analytics, and automated interventions to minimize air pollution and improve public health.
Studies have highlighted the importance of ICT in urban sustainability with big data, IoT, cloud services, smartphones and AI, including UAQM functions such as; setting policies, monitoring, in identifying emission sources, prediction, health assessment, dissemination and citizen participation.
Did you know this? Cities offer economic opportunities, but they also come with environmental challenges. One of the earliest major air pollution events was the London Smog of 1952, which led to over 4,000 deaths. Since then, scientists, policymakers, and governments have recognized air pollution as a serious risk to human health and have worked to address it (Stern, 1968).
Smart Traffic Management to Reduce Emissions
Traffic congestion is a major contributor to urban air pollution, with vehicle emissions accounting for up to 60% of air pollution in cities. Urban computing enables smart traffic management systems that use AI-driven analytics and IoT sensors to optimize traffic flow and reduce congestion.
Smart traffic management systems help reduce pollution by optimizing routes, promoting ridesharing, and improving parking solutions. According to a research, these systems could cut 205 million metric tons of CO₂ emissions by 2027. This shows how smart technology can significantly lower our carbon footprint and support sustainability.
Adaptive traffic signals adjust in real-time based on traffic volume and air quality levels, reducing idling emissions.
Intelligent route optimization helps public transport and delivery vehicles take the least polluted paths.
Vehicle emission monitoring using ANPR (Automatic Number Plate Recognition) and remote sensors allows cities to enforce low-emission zones effectively.
By integrating smart traffic systems with air quality sensors, cities can significantly lower NO₂, PM, and CO emissions, contributing to cleaner urban environments.
AI-Powered Pollution Forecasting Systems
AI and machine learning (ML) models enhance air quality forecasting by analyzing historical pollution trends, meteorological data, and emission patterns. These models process large-scale environmental data from IoT sensors, satellites, and weather stations to predict pollution spikes with high accuracy.
AI and machine learning are playing a big role in monitoring and predicting air quality by improving accuracy and efficiency. Advanced models like LSTM and GRU, which are types of neural networks, have been effective in predicting nitrogen dioxide (NO₂) levels, performing better than traditional methods despite some challenges with data quality.
By combining IoT devices with cloud-based AI models, like the BO-HyTS model (which merges SARIMA and LSTM), air quality forecasts have become more accurate. This approach helps capture both simple and complex data patterns, leading to better AQI predictions.
Deep learning algorithms analyze real-time pollutant dispersion and wind patterns to forecast air quality levels up to 72 hours in advance.
Predictive analytics help city officials implement proactive measures like restricting industrial emissions or controlling vehicular traffic before pollution peaks.
AI models integrated with urban planning tools assist in identifying long-term pollution mitigation strategies, such as optimal placement of green spaces or air filtration zones.
Public Air Quality Alerts and Citizen Engagement
Raising public awareness and empowering citizens with real-time air quality data is a key aspect of smart city pollution management. Urban computing enables:
Mobile apps and smart billboards to display real-time AQI (Air Quality Index) levels.
Personalized pollution exposure alerts, advising individuals with respiratory conditions to take precautions.
Crowdsourced pollution mapping, where citizens report pollution sources using geo-tagged mobile applications.
By integrating public engagement like collective effort, not just government action. Smart cities and communities like IAQN play a key role by raising awareness, uniting experts, and driving action. Real-time air quality monitoring, public engagement, and knowledge-sharing through conferences help turn insights into solutions, creating cleaner, healthier cities for all with real-time air quality monitoring, smart cities can foster community-driven action for air pollution mitigation.
Challenges and Future Trends in Smart Air Quality Management
Smart air quality management relies on IoT sensors, AI, and big data analytics, but challenges in data security, sensor accuracy, and technology standardization remain barriers to effective implementation. Addressing these issues is crucial for the future of urban air pollution control.
Data Privacy and Security Concerns in Smart Monitoring
Air quality monitoring generates vast amounts of real-time environmental data, often stored on cloud-based platforms. However, data security and privacy risks arise due to:
Unauthorized access and cyber threats, which can manipulate or compromise pollution data.
Lack of clear data governance policies, leading to potential misuse of sensitive environmental information.
Public concern over surveillance, as monitoring systems often track vehicular emissions and industrial activities.
Ensuring Accuracy and Standardization of Sensor Data
IoT-based air quality sensors vary in sensitivity, calibration, and maintenance, leading to inconsistencies in pollution data. Common challenges include:
Sensor drift and calibration errors, affecting long-term data reliability.
Variability in sensor quality, as different manufacturers follow different standards.
Lack of global calibration protocols, making data comparison between cities difficult.
To overcome this, smart cities must adopt standardized sensor calibration methods, AI-based data correction algorithms, and real-time quality control measures to enhance data accuracy.
Infrastructure and Maintenance
Establishing an extensive IoT sensor network requires significant investment in hardware, connectivity, and cloud storage. Deploying high-density air quality sensors in urban areas demands robust infrastructure, but sensor maintenance, calibration, and data transmission costs pose financial and technical hurdles. Additionally, environmental factors like extreme weather conditions can degrade sensor performance, requiring frequent maintenance and recalibration to ensure data accuracy.
Interoperability
With multiple vendors supplying air quality sensors and platforms, ensuring seamless data integration is a challenge. Variability in communication protocols (LoRaWAN, NB-IoT, Wi-Fi, and 5G), data formats, and calibration techniques makes cross-platform compatibility difficult. Interoperability standards and open-source frameworks are essential to integrate real-time data from diverse networks into a unified system.
Regulatory & Ethical Challenges in Urban Computing
Policy Gaps: Bridging Technology & Regulation:
EU Air Quality Directive: Sets emission reduction targets, but urban computing adoption varies by city funding.
US EPA AirNow Program: Uses sensor networks, but struggles with real-time AI-based forecasting.
India’s NCAP: Focuses on manual pollution control, with limited AI-driven interventions.
Policy Challenge:
Governments rely on stationary reference monitors, but low-cost sensor data isn't always considered in official policies.
Solution: Calibration frameworks where IoT data is benchmarked against government monitors.
Ethical & Privacy Concerns in Air Quality Monitoring
Citizen-Level Air Exposure Monitoring: AI can track individual exposure to pollution, raising privacy issues.
Bias in AI Predictions: Urban datasets overrepresent commercial zones while ignoring marginalized communities.
Solution: Decentralized & Blockchain-Based Air Quality Data
Ensures data integrity and prevents tampering in environmental monitoring reports.
IBM’s Blockchain Air Quality Initiative provides tamper-proof pollution records for policy decisions.
Future Innovations: AI and Machine Learning in Pollution Control
Emerging technologies like AI and machine learning are revolutionizing air pollution management by:
Predicting pollution trends based on historical and real-time data.
Optimizing industrial emission controls by detecting anomalies in factory pollution levels.
Enhancing traffic management, using AI to reduce congestion and minimize vehicular emissions.
Key Innovations to Watch
Innovation | Impact on Air Quality |
Edge AI for Pollution Sensors | Faster real-time decision-making at sensor level, reducing latency. |
AI-Driven Smart Traffic Systems | Reduces vehicle emissions by dynamically adjusting traffic flow. |
Blockchain for Secure Data Sharing | Ensures transparent air quality reporting and prevents data manipulation. |
5G-Enabled Sensor Networks | Ultra-fast data transmission for hyperlocal monitoring. |
Conclusion
Urban computing is changing the way cities manage air pollution by using IoT, AI, and big data to monitor and improve air quality in real time. From smart traffic control to AI-powered pollution predictions, these technologies help cities reduce emissions and protect public health.
But there are still challenges, sensor accuracy, data security, infrastructure costs, and system compatibility need improvement. The future looks promising with better calibration methods, secure blockchain data storage, and AI-powered self-correcting sensors, making air quality monitoring more reliable and scalable.
Looking ahead, edge computing will allow faster data analysis, while AI will enhance pollution forecasting and help cities take preventive actions. By combining technology with smart policies, cities can create cleaner, healthier environments for everyone.
Alongside these advancements, communities like the Indian Air Quality Network (IAQN) play a vital role in raising awareness, connecting experts, and driving action for air pollution mitigation. Through collaborative efforts between researchers, policymakers, and the public, we can accelerate the shift towards cleaner, smarter cities.
With innovation and community-driven efforts, achieving better air quality is within reach. The journey has just begun, and the future of clean air is powered by smart technology and collective action.
Comentários